Researchers use fitness tracker data and machine learning to detect mood swings in bipolar disorder
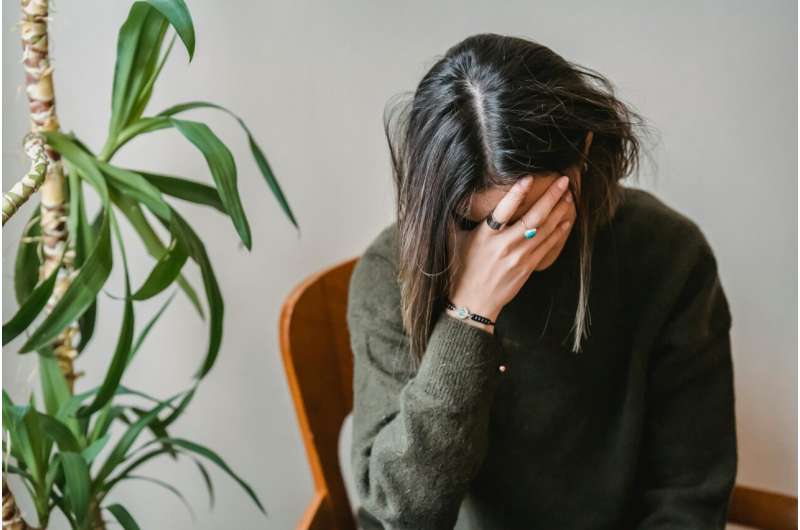
Credit: Liza Summer from Pexels
Study shows exercise therapist can identify mood swings in bipolar disorder to help guide treatment
Researchers at Brigham and Women’s Hospital, a founding member of the Mass General Brigham health system, tested whether data collected from a fitness tracker could be used to accurately detect emotional events in people who with bipolar disorder. Their findings, published in Scandinavian Psychiatric Journalshow that it is possible to detect periods of time when patients with bipolar disorder experience depression or mania with high accuracy using data from exercise tracking devices.
“Many people travel with personal digital devices such as smartphones and smartwatches that capture daily information that can inform psychotherapy. Our goal was to use that information to identify participants. those studied who were diagnosed with bipolar disorder had more emotional episodes,” said corresponding author Jessica Lipschitz, Ph.D., a researcher at Brigham’s Department of Psychiatry.
“In the future, our hope is that machine learning techniques like ours can help patient care teams respond more quickly to new or ongoing events in order to reduce negative impact.”
Bipolar disorder (BD) is a chronic mental illness characterized by extreme mood swings, including depression, anxiety, and hypomania followed by periods of relaxation. Identification and treatment of new and persistent mood episodes is important to reduce the impact of BD on patients’ lives. Although previous research has shown that personal digital devices can accurately detect emotional events, previous studies have not used methods designed for widespread use in clinical settings.
As an implementation scientist, Lipschitz, along with his colleagues, are focused on applying methods that can be widely used in clinical practice. In particular, they used their own commercially available digital tools, limited data filtering, and comprehensive and non-invasive data collection. Using a new type of machine learning algorithm, they were able to recognize the clinically significant symptoms of depression with 80.1% accuracy and the clinically significant symptoms of mania with 89.1% accuracy.
The researchers say, “Overall, the results move the field a step toward individualized algorithms that are appropriate for the entire patient population, rather than just those with high performance, to find tools that special, or willingness to share offensive information.”
Their next step is to apply these predictive algorithms to routine care where they can be used to improve BD treatment by notifying doctors when their patients are having depressive or manic episodes. between scheduled meetings. Researchers are also working to extend this work to major depressive disorder.
Additional information:
Jessica M. Lipschitz et al, Digital phenotyping in bipolar disorder: Using longitudinal Fitbit data and individual machine learning to predict mood symptoms, Scandinavian Psychiatric Journal (2024). DOI: 10.1111/acps.13765
Provided by Brigham and Women’s Hospital
Excerpt: Researchers use exercise tracker data and machine learning to predict mood swings in bipolar disorder (2024, November 25) Retrieved November 26, 2024 from https://medicalxpress.com/news /2024-11-tracker-machine-bipolar-disorder-mood. html
This document is subject to copyright. Except for any legitimate activity for the purpose of private study or research, no part may be reproduced without written permission. Content is provided for informational purposes only.
#Researchers #fitness #tracker #data #machine #learning #detect #mood #swings #bipolar #disorder